What is Retrieval Augmented Generation?
What Retrieval Augmented Generation (RAG) is, how it can make large language models hallucinate less and how we are using this on Retinello.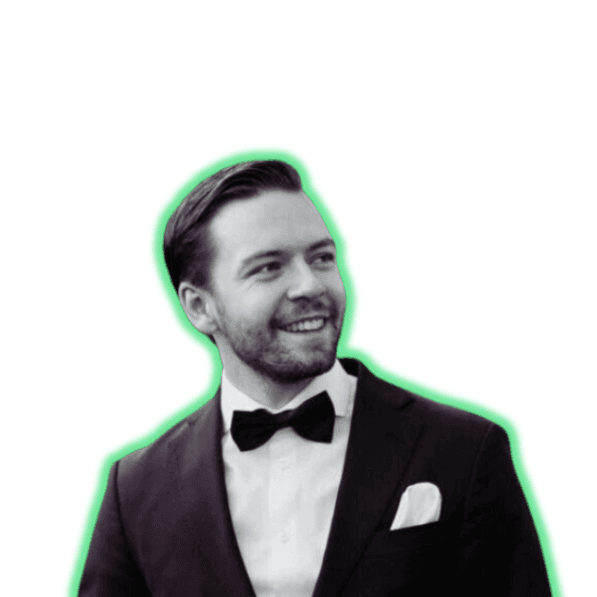
Daniel Kouznetsov
Published: 2023-12-27Large language models (LLMs) such as ChatGPT has a tendency to sometimes, very confidently, state wrongful statements and facts that does not align with reality. This is a problem that is inherent in LLMs. When using AI in education this is a very obvious problem, students should learn facts and not misinformation. Is there a solution to the problem? As of now no one has solved the problem fully but one can drastically reduce the amount of hallucinations from LLMs. One such solution is using Retrieval Augmented Generation (RAG).
Retrieval Augmented Generation at it’s very core
At it’s very core RAG is really simple: from a user perspective it’s just like copy pasting relevant data into your chat message. Let’s say that you have a niche subject that the LLM hasn’t been trained on, it’s going to do it’s best to find a solution for you that it thinks is correct but might be terribly wrong. What you could do to mitigate this is to provide the AI with context, i.e., text relevant to what you are asking it about. As efficient human beings we don’t want to do this manually! So what RAG is doing is it is finding relevant paragraphs from your documents and copy pastes this into your chat message.
This is RAG at it’s very core but it does not stop there. You can do way more advanced stuff with RAG to make sure that you really do get the most relevant text retrieved. This will be further discussed in later articles, be sure to stick around and sign up to our newsletter (below!).
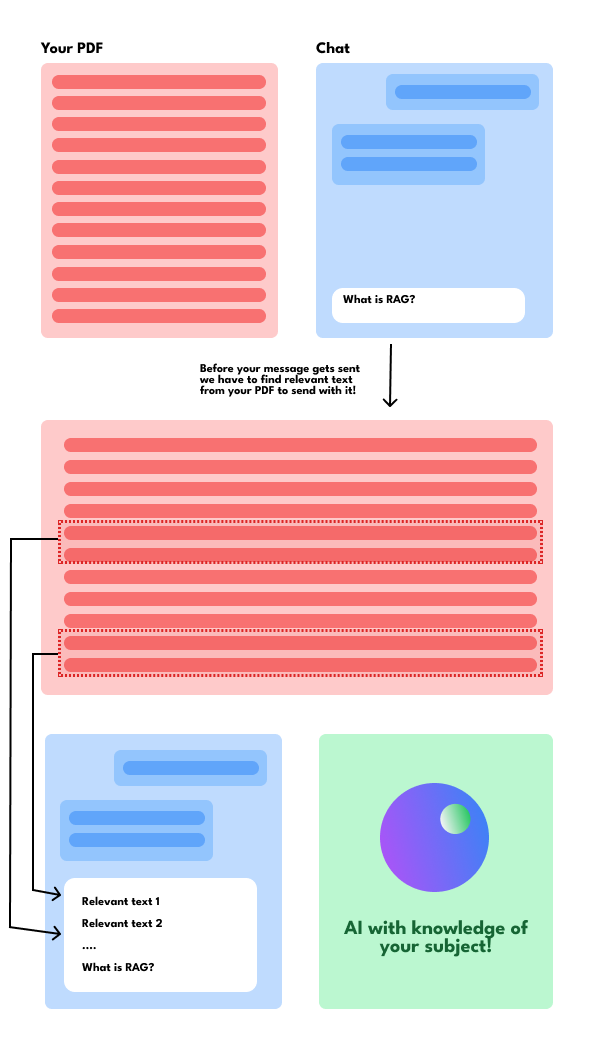
How do we use RAG on Retinello?
On Retinello premium users can upload and save their documents. These will then be transformed into vectors and stored in a vector database. Without going into much technical detail: vectors are used to calculate the distance between two different pieces of text to make sure that you get the most relevant text retrieved. OK so it’s stored in a vector database, what next?
You can have a normal “chat with pdf”-style of service where you just chat directly to your document, where every single chat message can be turned into flashcards! You can also attach your documents to your decks. Why? It is because when you study using interactive learning you want to keep the model informed of what you are studying, so when you have a document attached to your deck it will do RAG for you in the background when you’re studying using interactive learning!